Jacob Chanyeol Choi
Nov 5, 2024
Trusted Data Sources, Evaluation Agents, Interactive Validation: ensuring accuracy & trust in investment research.
Investment research is one of the most meticulous disciplines in the financial world. It requires not only the strategic amassment of data, but also the skill of reasoning through complex and often ambiguous information to predict trends and long term consequences. Analysts are the backbone of this process, applying their expertise to mounds of data to arrive at actionable insights. Given the complexity of their tasks, it’s clear that no single technology can address all the challenges analysts face. This is why Linq Alpha employs a combination of complex technologies to uniquely address this problem:
Context-aware hybrid search to enhance domain-specific search capabilities.
Finance-specific embeddings to achieve the last mile of accuracy.
Yet, even with these technologies, the task of ensuring accuracy in AI-driven research extends beyond just algorithms and models. This is especially true in mind of hallucinations, addressing which requires a nuanced and comprehensive approach. We explore the standing issues and how we address them below.
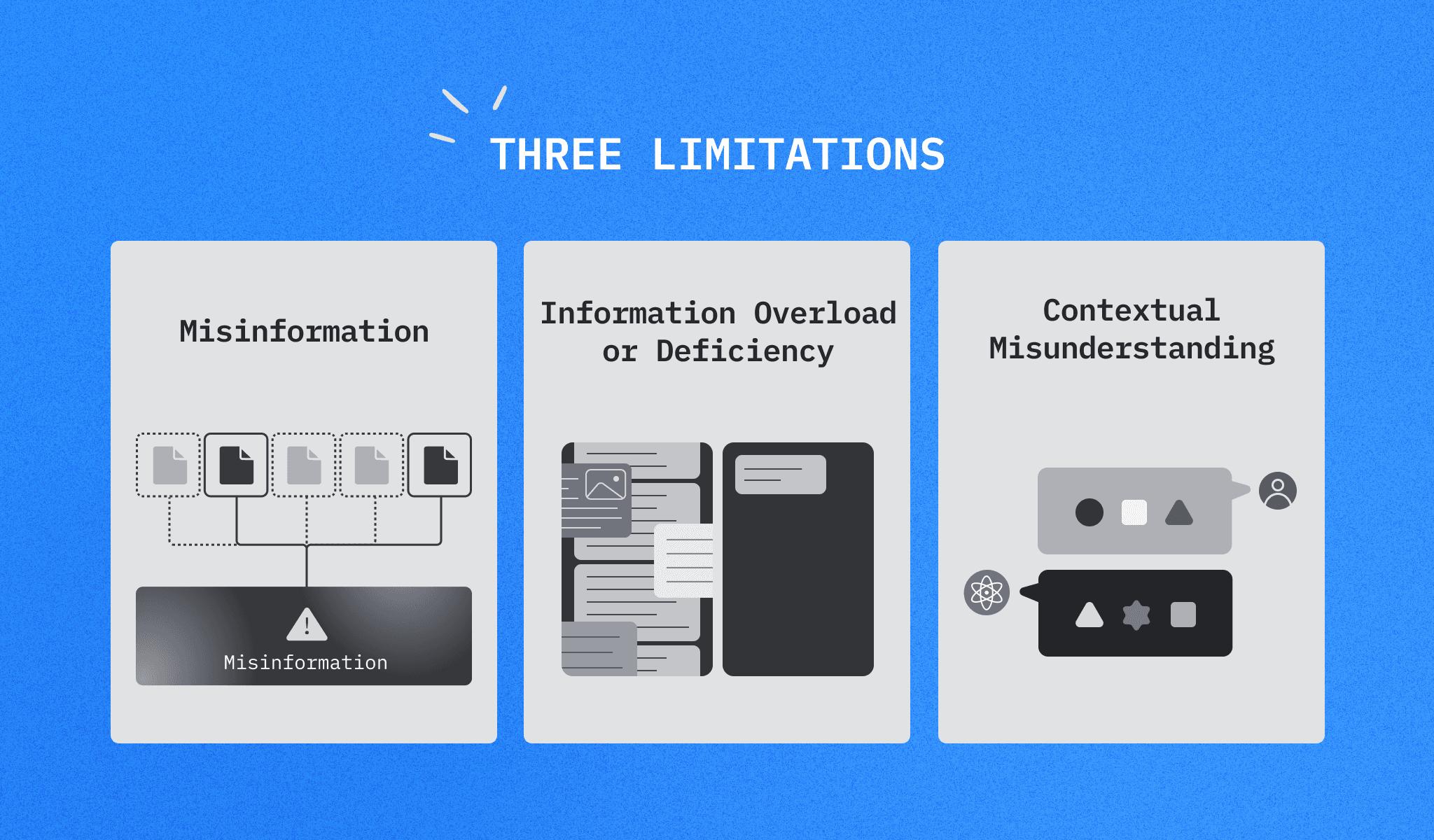
The Issue of Hallucinations
Hallucinations in AI, pose significant challenges in the context of financial research. Just as humans can feel overwhelmed or perplexed by incomplete or vast amounts of information, AI models can also falter. To ensure Linq Alpha, our LLM-based model, effectively navigates such contexts we continuously refine our solution with a focus on addressing the following types of hallucinations.
Misinformation: When legitimate, high-quality information is unavailable, the AI might generate responses that are factually incorrect or misleading. Just as a human might draw incorrect conclusions from unreliable sources, AI can also err when the information it relies on is flawed.
Information Overload or Deficiency: When faced with conflicting information or insufficient data, the AI, like a person overwhelmed by contradictory news or lacking enough details, may struggle to make accurate judgments.
Contextual Misunderstanding: When the AI fails to fully comprehend the user’s context, it can produce misaligned or irrelevant responses, similar to how a person might misunderstand a situation without complete information.
Recognizing these challenges, Linq Alpha employs three critical guardrails to ensure that the information it provides is not only accurate but also contextually appropriate and actionable, in the form of Trusted Data Sources, Evaluation Agents, and Interactive Validation.

Trusted Data Sources
In response to the challenge of misinformation, Linq Alpha is dedicated to exclusively utilizing Trusted Data Sources. . This first guardrail is especially critical when legitimate, high-quality data is sparse or difficult to find, as Linq Alpha’s proprietary data ensures the AI can still deliver accurate, context-specific insights even in challenging queries. By sourcing, curating, and utilizing only the most trustworthy information, Linq Alpha minimizes the risk of misinformation
Evaluation Agents
The second guardrail involves Evaluation Agents, developed and verified by experienced ex-analysts within the company. These agents prevent information overload or deficiencies, by performing rigorous checks to ensure that the AI’s responses are based on complete and accurate information. They assess whether the data is sufficient or if additional cross-referenced sources are needed. Furthermore, in cases where the AI encounters an overload of data, these agents help prioritize the most authentic and relevant information, ensuring that less critical details do not cloud the final output. This layered verification process is essential in maintaining the high standards of accuracy that financial analysts demand.
Interactive Validation
Lastly, the third guardrail addresses the issue of contextual misunderstanding via Interactive Validation. By allowing users to validate the AI’s output through citations and links to reference materials, users can immediately verify that the information is relevant to their specific needs. This validation process, combined with Linq Alpha’s support of multi-turn conversations, allows the AI to develop a term memory of the user’s needs over time, leading to increasingly contextually relevant responses.
Bridging the Gap Between Technology and Trust
Having been in this field for two years since its inception, we realized that GenAI cannot be perfected overnight. Hence, we’ve established a robust evaluation system characterized by three key guardrails. This iterative process ensures that our AI is both accurate and reliable.
The application of our guardrails—Trusted Data Sources, Evaluation Agents, and Interactive Validation—complements the advanced technologies we’ve discussed in earlier posts. While those technologies are vital for driving the precision of Linq Alpha, our guardrails provide the necessary framework to ensure that such precision translates into real-world accuracy and trust.
At Linq, we recognize the complexity and multi-faceted nature of fundamental research. By continuously refining and enhancing our guardrails, we are committed to bringing Linq Alpha closer to the goal of becoming a valuable ally for buy-side analysts, delivering accurate and reliable insights that support even their most critical decision-making processes.
👉 Curious about how Linq Alpha’s tailored search solutions can enhance your financial research? please join our waitlist.